411 lines
15 KiB
Markdown
411 lines
15 KiB
Markdown
# 基于图神经网络的异构图表示学习和推荐算法研究
|
||
|
||
## 目录结构
|
||
|
||
```
|
||
GNN-Recommendation/
|
||
gnnrec/ 算法模块顶级包
|
||
hge/ 异构图表示学习模块
|
||
kgrec/ 基于图神经网络的推荐算法模块
|
||
data/ 数据集目录(已添加.gitignore)
|
||
model/ 模型保存目录(已添加.gitignore)
|
||
img/ 图片目录
|
||
academic_graph/ Django项目模块
|
||
rank/ Django应用
|
||
manage.py Django管理脚本
|
||
```
|
||
|
||
## 安装依赖
|
||
|
||
Python 3.7
|
||
|
||
### CUDA 11.0
|
||
|
||
```shell
|
||
pip install -r requirements_cuda.txt
|
||
```
|
||
|
||
### CPU
|
||
|
||
```shell
|
||
pip install -r requirements.txt
|
||
```
|
||
|
||
## 异构图表示学习(附录)
|
||
|
||
基于对比学习的关系感知异构图神经网络(Relation-aware Heterogeneous Graph Neural Network with Contrastive Learning, RHCO)
|
||
|
||

|
||
|
||
### 实验
|
||
|
||
见 [readme](gnnrec/hge/readme.md)
|
||
|
||
## 基于图神经网络的推荐算法(附录)
|
||
|
||
基于图神经网络的学术推荐算法(Graph Neural Network based Academic Recommendation Algorithm, GARec)
|
||
|
||

|
||
|
||
### 实验
|
||
|
||
见 [readme](gnnrec/kgrec/readme.md)
|
||
|
||
## Django 配置
|
||
|
||
### MySQL 数据库配置
|
||
|
||
1. 创建数据库及用户
|
||
|
||
```sql
|
||
CREATE DATABASE academic_graph CHARACTER SET utf8mb4;
|
||
CREATE USER 'academic_graph'@'%' IDENTIFIED BY 'password';
|
||
GRANT ALL ON academic_graph.* TO 'academic_graph'@'%';
|
||
```
|
||
|
||
2. 在根目录下创建文件.mylogin.cnf
|
||
|
||
```ini
|
||
[client]
|
||
host = x.x.x.x
|
||
port = 3306
|
||
user = username
|
||
password = password
|
||
database = database
|
||
default-character-set = utf8mb4
|
||
```
|
||
|
||
3. 创建数据库表
|
||
|
||
```shell
|
||
python manage.py makemigrations --settings=academic_graph.settings.prod rank
|
||
python manage.py migrate --settings=academic_graph.settings.prod
|
||
```
|
||
|
||
4. 导入 oag-cs 数据集
|
||
|
||
```shell
|
||
python manage.py loadoagcs --settings=academic_graph.settings.prod
|
||
```
|
||
|
||
注:由于导入一次时间很长(约 9 小时),为了避免中途发生错误,可以先用 data/oag/test 中的测试数据调试一下
|
||
|
||
### 拷贝静态文件
|
||
|
||
```shell
|
||
python manage.py collectstatic --settings=academic_graph.settings.prod
|
||
```
|
||
|
||
### 启动 Web 服务器
|
||
|
||
```shell
|
||
export SECRET_KEY=xxx
|
||
python manage.py runserver --settings=academic_graph.settings.prod 0.0.0.0:8000
|
||
```
|
||
|
||
### 系统截图
|
||
|
||
搜索论文
|
||
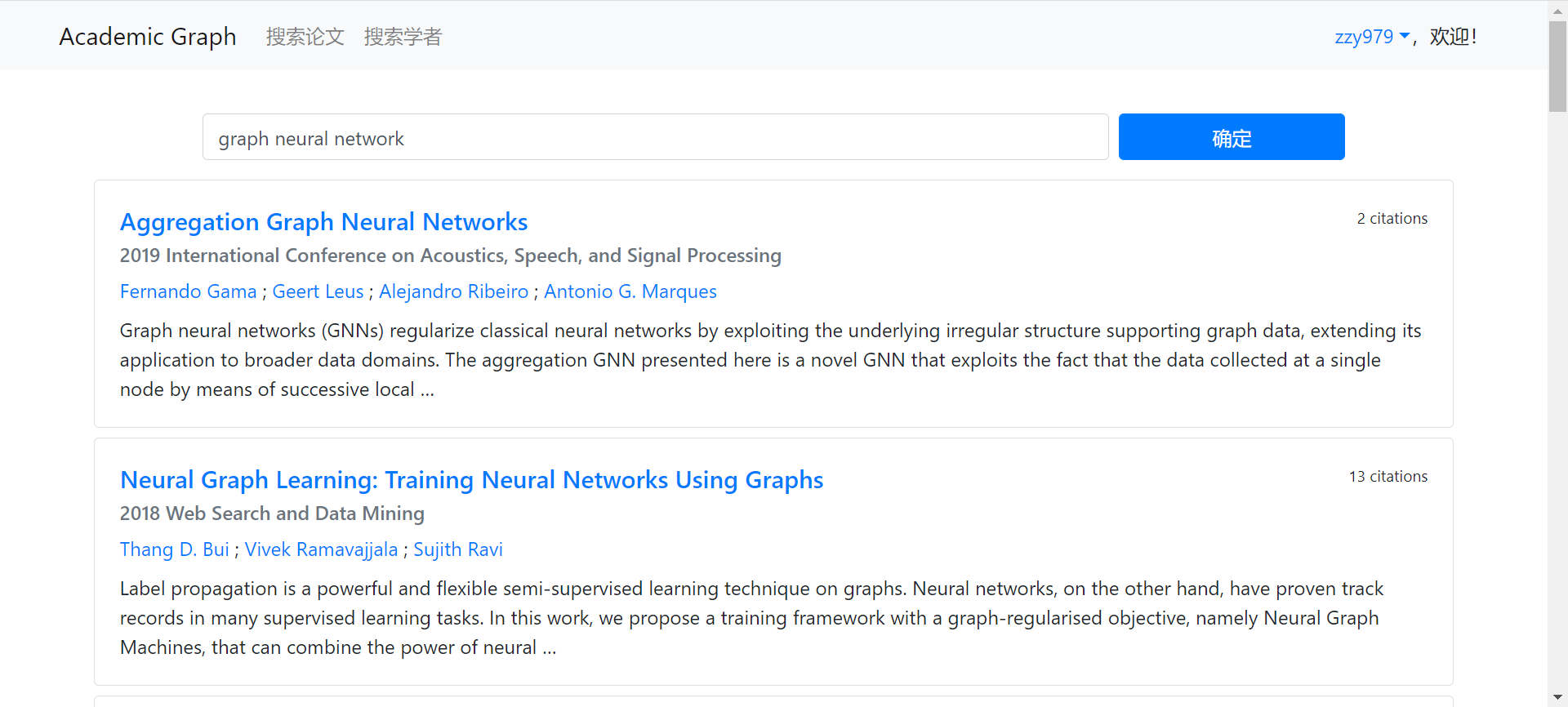
|
||
|
||
论文详情
|
||
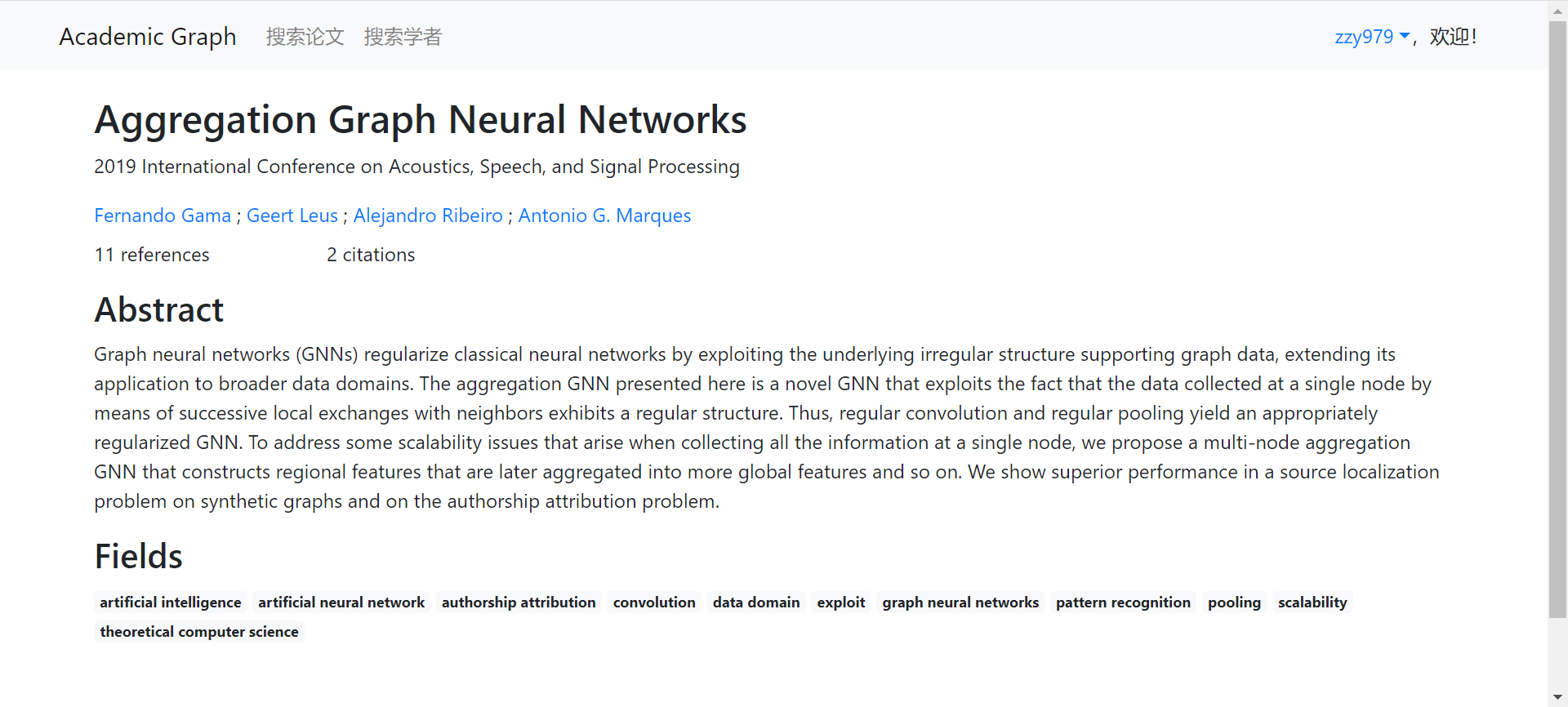
|
||
|
||
搜索学者
|
||

|
||
|
||
学者详情
|
||
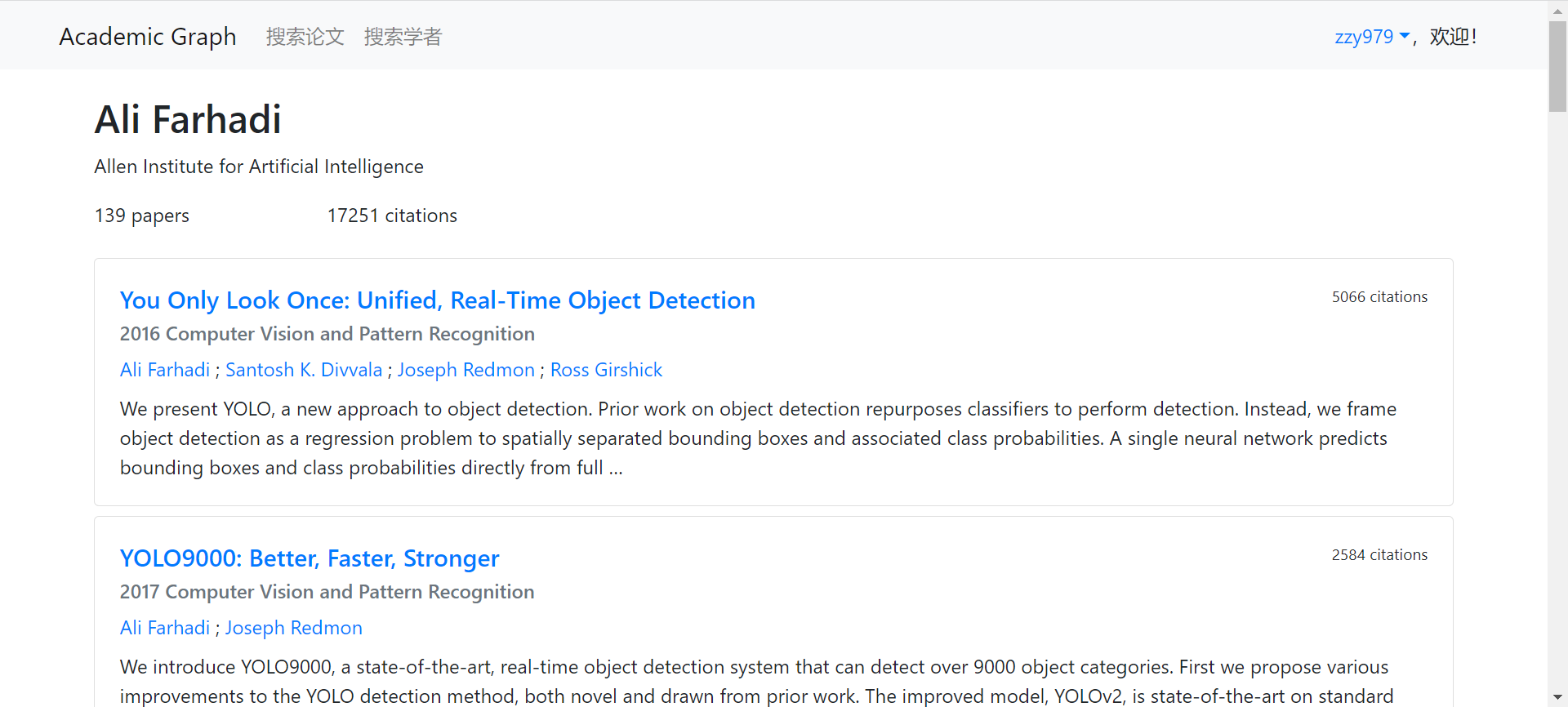
|
||
|
||
## 附录
|
||
|
||
### 基于图神经网络的推荐算法
|
||
|
||
#### 数据集
|
||
|
||
oag-cs - 使用 OAG 微软学术数据构造的计算机领域的学术网络(见 [readme](data/readme.md))
|
||
|
||
#### 预训练顶点嵌入
|
||
|
||
使用 metapath2vec(随机游走 +word2vec)预训练顶点嵌入,作为 GNN 模型的顶点输入特征
|
||
|
||
1. 随机游走
|
||
|
||
```shell
|
||
python -m gnnrec.kgrec.random_walk model/word2vec/oag_cs_corpus.txt
|
||
```
|
||
|
||
2. 训练词向量
|
||
|
||
```shell
|
||
python -m gnnrec.hge.metapath2vec.train_word2vec --size=128 --workers=8 model/word2vec/oag_cs_corpus.txt model/word2vec/oag_cs.model
|
||
```
|
||
|
||
#### 召回
|
||
|
||
使用微调后的 SciBERT 模型(见 [readme](data/readme.md) 第 2 步)将查询词编码为向量,与预先计算好的论文标题向量计算余弦相似度,取 top k
|
||
|
||
```shell
|
||
python -m gnnrec.kgrec.recall
|
||
```
|
||
|
||
召回结果示例:
|
||
|
||
graph neural network
|
||
|
||
```
|
||
0.9629 Aggregation Graph Neural Networks
|
||
0.9579 Neural Graph Learning: Training Neural Networks Using Graphs
|
||
0.9556 Heterogeneous Graph Neural Network
|
||
0.9552 Neural Graph Machines: Learning Neural Networks Using Graphs
|
||
0.9490 On the choice of graph neural network architectures
|
||
0.9474 Measuring and Improving the Use of Graph Information in Graph Neural Networks
|
||
0.9362 Challenging the generalization capabilities of Graph Neural Networks for network modeling
|
||
0.9295 Strategies for Pre-training Graph Neural Networks
|
||
0.9142 Supervised Neural Network Models for Processing Graphs
|
||
0.9112 Geometrically Principled Connections in Graph Neural Networks
|
||
```
|
||
|
||
recommendation algorithm based on knowledge graph
|
||
|
||
```
|
||
0.9172 Research on Video Recommendation Algorithm Based on Knowledge Reasoning of Knowledge Graph
|
||
0.8972 An Improved Recommendation Algorithm in Knowledge Network
|
||
0.8558 A personalized recommendation algorithm based on interest graph
|
||
0.8431 An Improved Recommendation Algorithm Based on Graph Model
|
||
0.8334 The Research of Recommendation Algorithm based on Complete Tripartite Graph Model
|
||
0.8220 Recommendation Algorithm based on Link Prediction and Domain Knowledge in Retail Transactions
|
||
0.8167 Recommendation Algorithm Based on Graph-Model Considering User Background Information
|
||
0.8034 A Tripartite Graph Recommendation Algorithm Based on Item Information and User Preference
|
||
0.7774 Improvement of TF-IDF Algorithm Based on Knowledge Graph
|
||
0.7770 Graph Searching Algorithms for Semantic-Social Recommendation
|
||
```
|
||
|
||
scholar disambiguation
|
||
|
||
```
|
||
0.9690 Scholar search-oriented author disambiguation
|
||
0.9040 Author name disambiguation in scientific collaboration and mobility cases
|
||
0.8901 Exploring author name disambiguation on PubMed-scale
|
||
0.8852 Author Name Disambiguation in Heterogeneous Academic Networks
|
||
0.8797 KDD Cup 2013: author disambiguation
|
||
0.8796 A survey of author name disambiguation techniques: 2010–2016
|
||
0.8721 Who is Who: Name Disambiguation in Large-Scale Scientific Literature
|
||
0.8660 Use of ResearchGate and Google CSE for author name disambiguation
|
||
0.8643 Automatic Methods for Disambiguating Author Names in Bibliographic Data Repositories
|
||
0.8641 A brief survey of automatic methods for author name disambiguation
|
||
```
|
||
|
||
### 精排
|
||
|
||
#### 构造 ground truth
|
||
|
||
(1)验证集
|
||
|
||
从 AMiner 发布的 [AI 2000 人工智能全球最具影响力学者榜单](https://www.aminer.cn/ai2000) 抓取人工智能 20 个子领域的 top 100 学者
|
||
|
||
```shell
|
||
pip install scrapy>=2.3.0
|
||
cd gnnrec/kgrec/data/preprocess
|
||
scrapy runspider ai2000_crawler.py -a save_path=/home/zzy/GNN-Recommendation/data/rank/ai2000.json
|
||
```
|
||
|
||
与 oag-cs 数据集的学者匹配,并人工确认一些排名较高但未匹配上的学者,作为学者排名 ground truth 验证集
|
||
|
||
```shell
|
||
export DJANGO_SETTINGS_MODULE=academic_graph.settings.common
|
||
export SECRET_KEY=xxx
|
||
python -m gnnrec.kgrec.data.preprocess.build_author_rank build-val
|
||
```
|
||
|
||
(2)训练集
|
||
|
||
参考 AI 2000 的计算公式,根据某个领域的论文引用数加权求和构造学者排名,作为 ground truth 训练集
|
||
|
||
计算公式:
|
||
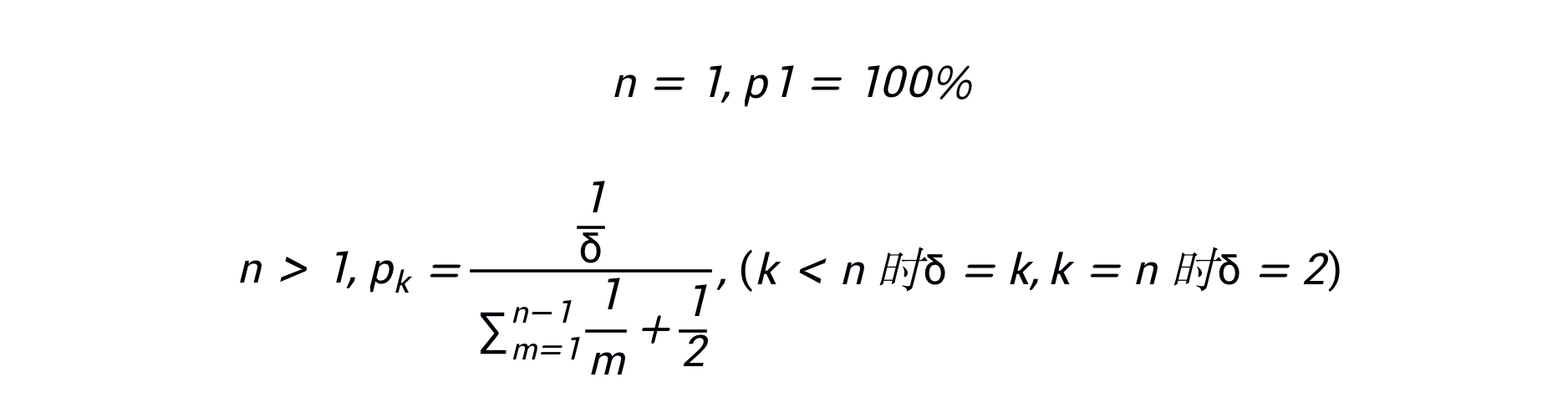
|
||
即:假设一篇论文有 n 个作者,第 k 作者的权重为 1/k,最后一个视为通讯作者,权重为 1/2,归一化之后计算论文引用数的加权求和
|
||
|
||
```shell
|
||
python -m gnnrec.kgrec.data.preprocess.build_author_rank build-train
|
||
```
|
||
|
||
(3)评估 ground truth 训练集的质量
|
||
|
||
```shell
|
||
python -m gnnrec.kgrec.data.preprocess.build_author_rank eval
|
||
```
|
||
|
||
```
|
||
nDGC@100=0.2420 Precision@100=0.1859 Recall@100=0.2016
|
||
nDGC@50=0.2308 Precision@50=0.2494 Recall@50=0.1351
|
||
nDGC@20=0.2492 Precision@20=0.3118 Recall@20=0.0678
|
||
nDGC@10=0.2743 Precision@10=0.3471 Recall@10=0.0376
|
||
nDGC@5=0.3165 Precision@5=0.3765 Recall@5=0.0203
|
||
```
|
||
|
||
(4)采样三元组
|
||
|
||
从学者排名训练集中采样三元组(t, ap, an),表示对于领域 t,学者 ap 的排名在 an 之前
|
||
|
||
```shell
|
||
python -m gnnrec.kgrec.data.preprocess.build_author_rank sample
|
||
```
|
||
|
||
#### 训练 GNN 模型
|
||
|
||
```shell
|
||
python -m gnnrec.kgrec.train model/word2vec/oag-cs.model model/garec_gnn.pt data/rank/author_embed.pt
|
||
```
|
||
|
||
## 异构图表示学习
|
||
|
||
### 数据集
|
||
|
||
* [ACM](https://github.com/liun-online/HeCo/tree/main/data/acm) - ACM 学术网络数据集
|
||
* [DBLP](https://github.com/liun-online/HeCo/tree/main/data/dblp) - DBLP 学术网络数据集
|
||
* [ogbn-mag](https://ogb.stanford.edu/docs/nodeprop/#ogbn-mag) - OGB 提供的微软学术数据集
|
||
* [oag-venue](../kgrec/data/venue.py) - oag-cs 期刊分类数据集
|
||
|
||
| 数据集 | 顶点数 | 边数 | 目标顶点 | 类别数 |
|
||
| --------- | ------- | -------- | -------- | ------ |
|
||
| ACM | 11246 | 34852 | paper | 3 |
|
||
| DBLP | 26128 | 239566 | author | 4 |
|
||
| ogbn-mag | 1939743 | 21111007 | paper | 349 |
|
||
| oag-venue | 4235169 | 34520417 | paper | 360 |
|
||
|
||
### Baselines
|
||
|
||
* [R-GCN](https://arxiv.org/pdf/1703.06103)
|
||
* [HGT](https://arxiv.org/pdf/2003.01332)
|
||
* [HGConv](https://arxiv.org/pdf/2012.14722)
|
||
* [R-HGNN](https://arxiv.org/pdf/2105.11122)
|
||
* [C&S](https://arxiv.org/pdf/2010.13993)
|
||
* [HeCo](https://arxiv.org/pdf/2105.09111)
|
||
|
||
#### R-GCN (full batch)
|
||
|
||
```shell
|
||
python -m gnnrec.hge.rgcn.train --dataset=acm --epochs=10
|
||
python -m gnnrec.hge.rgcn.train --dataset=dblp --epochs=10
|
||
python -m gnnrec.hge.rgcn.train --dataset=ogbn-mag --num-hidden=48
|
||
python -m gnnrec.hge.rgcn.train --dataset=oag-venue --num-hidden=48 --epochs=30
|
||
```
|
||
|
||
(使用 minibatch 训练准确率就是只有 20% 多,不知道为什么)
|
||
|
||
#### 预训练顶点嵌入
|
||
|
||
使用 metapath2vec(随机游走 +word2vec)预训练顶点嵌入,作为 GNN 模型的顶点输入特征
|
||
|
||
```shell
|
||
python -m gnnrec.hge.metapath2vec.random_walk model/word2vec/ogbn-mag_corpus.txt
|
||
python -m gnnrec.hge.metapath2vec.train_word2vec --size=128 --workers=8 model/word2vec/ogbn-mag_corpus.txt model/word2vec/ogbn-mag.model
|
||
```
|
||
|
||
#### HGT
|
||
|
||
```shell
|
||
python -m gnnrec.hge.hgt.train_full --dataset=acm
|
||
python -m gnnrec.hge.hgt.train_full --dataset=dblp
|
||
python -m gnnrec.hge.hgt.train --dataset=ogbn-mag --node-embed-path=model/word2vec/ogbn-mag.model --epochs=40
|
||
python -m gnnrec.hge.hgt.train --dataset=oag-venue --node-embed-path=model/word2vec/oag-cs.model --epochs=40
|
||
```
|
||
|
||
#### HGConv
|
||
|
||
```shell
|
||
python -m gnnrec.hge.hgconv.train_full --dataset=acm --epochs=5
|
||
python -m gnnrec.hge.hgconv.train_full --dataset=dblp --epochs=20
|
||
python -m gnnrec.hge.hgconv.train --dataset=ogbn-mag --node-embed-path=model/word2vec/ogbn-mag.model
|
||
python -m gnnrec.hge.hgconv.train --dataset=oag-venue --node-embed-path=model/word2vec/oag-cs.model
|
||
```
|
||
|
||
#### R-HGNN
|
||
|
||
```shell
|
||
python -m gnnrec.hge.rhgnn.train_full --dataset=acm --num-layers=1 --epochs=15
|
||
python -m gnnrec.hge.rhgnn.train_full --dataset=dblp --epochs=20
|
||
python -m gnnrec.hge.rhgnn.train --dataset=ogbn-mag model/word2vec/ogbn-mag.model
|
||
python -m gnnrec.hge.rhgnn.train --dataset=oag-venue --epochs=50 model/word2vec/oag-cs.model
|
||
```
|
||
|
||
#### C&S
|
||
|
||
```shell
|
||
python -m gnnrec.hge.cs.train --dataset=acm --epochs=5
|
||
python -m gnnrec.hge.cs.train --dataset=dblp --epochs=5
|
||
python -m gnnrec.hge.cs.train --dataset=ogbn-mag --prop-graph=data/graph/pos_graph_ogbn-mag_t5.bin
|
||
python -m gnnrec.hge.cs.train --dataset=oag-venue --prop-graph=data/graph/pos_graph_oag-venue_t5.bin
|
||
```
|
||
|
||
#### HeCo
|
||
|
||
```shell
|
||
python -m gnnrec.hge.heco.train --dataset=ogbn-mag model/word2vec/ogbn-mag.model data/graph/pos_graph_ogbn-mag_t5.bin
|
||
python -m gnnrec.hge.heco.train --dataset=oag-venue model/word2vec/oag-cs.model data/graph/pos_graph_oag-venue_t5.bin
|
||
```
|
||
|
||
(ACM 和 DBLP 的数据来自 [https://github.com/ZZy979/pytorch-tutorial/tree/master/gnn/heco](https://github.com/ZZy979/pytorch-tutorial/tree/master/gnn/heco) ,准确率和 Micro-F1 相等)
|
||
|
||
#### RHCO
|
||
|
||
基于对比学习的关系感知异构图神经网络(Relation-aware Heterogeneous Graph Neural Network with Contrastive Learning, RHCO)
|
||
|
||
在 HeCo 的基础上改进:
|
||
|
||
* 网络结构编码器中的注意力向量改为关系的表示(类似于 R-HGNN)
|
||
* 正样本选择方式由元路径条数改为预训练的 HGT 计算的注意力权重、训练集使用真实标签
|
||
* 元路径视图编码器改为正样本图编码器,适配 mini-batch 训练
|
||
* Loss 增加分类损失,训练方式由无监督改为半监督
|
||
* 在最后增加 C&S 后处理步骤
|
||
|
||
ACM
|
||
|
||
```shell
|
||
python -m gnnrec.hge.hgt.train_full --dataset=acm --save-path=model/hgt/hgt_acm.pt
|
||
python -m gnnrec.hge.rhco.build_pos_graph_full --dataset=acm --num-samples=5 --use-label model/hgt/hgt_acm.pt data/graph/pos_graph_acm_t5l.bin
|
||
python -m gnnrec.hge.rhco.train_full --dataset=acm data/graph/pos_graph_acm_t5l.bin
|
||
```
|
||
|
||
DBLP
|
||
|
||
```shell
|
||
python -m gnnrec.hge.hgt.train_full --dataset=dblp --save-path=model/hgt/hgt_dblp.pt
|
||
python -m gnnrec.hge.rhco.build_pos_graph_full --dataset=dblp --num-samples=5 --use-label model/hgt/hgt_dblp.pt data/graph/pos_graph_dblp_t5l.bin
|
||
python -m gnnrec.hge.rhco.train_full --dataset=dblp --use-data-pos data/graph/pos_graph_dblp_t5l.bin
|
||
```
|
||
|
||
ogbn-mag(第 3 步如果中断可使用--load-path 参数继续训练)
|
||
|
||
```shell
|
||
python -m gnnrec.hge.hgt.train --dataset=ogbn-mag --node-embed-path=model/word2vec/ogbn-mag.model --epochs=40 --save-path=model/hgt/hgt_ogbn-mag.pt
|
||
python -m gnnrec.hge.rhco.build_pos_graph --dataset=ogbn-mag --num-samples=5 --use-label model/word2vec/ogbn-mag.model model/hgt/hgt_ogbn-mag.pt data/graph/pos_graph_ogbn-mag_t5l.bin
|
||
python -m gnnrec.hge.rhco.train --dataset=ogbn-mag --num-hidden=64 --contrast-weight=0.9 model/word2vec/ogbn-mag.model data/graph/pos_graph_ogbn-mag_t5l.bin model/rhco_ogbn-mag_d64_a0.9_t5l.pt
|
||
python -m gnnrec.hge.rhco.smooth --dataset=ogbn-mag model/word2vec/ogbn-mag.model data/graph/pos_graph_ogbn-mag_t5l.bin model/rhco_ogbn-mag_d64_a0.9_t5l.pt
|
||
```
|
||
|
||
oag-venue
|
||
|
||
```shell
|
||
python -m gnnrec.hge.hgt.train --dataset=oag-venue --node-embed-path=model/word2vec/oag-cs.model --epochs=40 --save-path=model/hgt/hgt_oag-venue.pt
|
||
python -m gnnrec.hge.rhco.build_pos_graph --dataset=oag-venue --num-samples=5 --use-label model/word2vec/oag-cs.model model/hgt/hgt_oag-venue.pt data/graph/pos_graph_oag-venue_t5l.bin
|
||
python -m gnnrec.hge.rhco.train --dataset=oag-venue --num-hidden=64 --contrast-weight=0.9 model/word2vec/oag-cs.model data/graph/pos_graph_oag-venue_t5l.bin model/rhco_oag-venue.pt
|
||
python -m gnnrec.hge.rhco.smooth --dataset=oag-venue model/word2vec/oag-cs.model data/graph/pos_graph_oag-venue_t5l.bin model/rhco_oag-venue.pt
|
||
```
|
||
|
||
消融实验
|
||
|
||
```shell
|
||
python -m gnnrec.hge.rhco.train --dataset=ogbn-mag --model=RHCO_sc model/word2vec/ogbn-mag.model data/graph/pos_graph_ogbn-mag_t5l.bin model/rhco_sc_ogbn-mag.pt
|
||
python -m gnnrec.hge.rhco.train --dataset=ogbn-mag --model=RHCO_pg model/word2vec/ogbn-mag.model data/graph/pos_graph_ogbn-mag_t5l.bin model/rhco_pg_ogbn-mag.pt
|
||
```
|
||
|
||
### 实验结果
|
||
|
||
[顶点分类](gnnrec/hge/result/node_classification.csv)
|
||
|
||
[参数敏感性分析](gnnrec/hge/result/param_analysis.csv)
|
||
|
||
[消融实验](gnnrec/hge/result/ablation_study.csv)
|